Introduction
As artificial intelligence (AI) models continue to improve and become more accessible, the harms stemming from their misuse also continue to grow. Some of the most heinous misuse includes nonconsensual intimate imagery (NCII) and child sexual abuse material (CSAM). This paper will discuss the latter in the context of US approaches and policies. As defined by Thorn, a nonprofit that builds technology to defend children from sexual abuse, CSAM “refers to the sexually explicit content involving a child.” Deepfake CSAM, or AI-generated CSAM (AIG-CSAM) specifically refers to CSAM that is fully or partially created using AI.
This paper will review a key misconception around AIG-CSAM, its harms, complicating facets, and various mitigating solutions.
Methodology
This paper uses two main sources to understand AIG-CSAM and is part of a larger research portfolio used to create the game, The Deepfake Files. First, we interviewed seventeen experts in the field to understand the ways in which deepfakes could be mitigated broadly. These experts included cybersecurity experts, computer science researchers, nonprofit leaders, and government employees and were primarily based in the United States. Interviews were confidential, semi-structured, and lasted approximately thirty minutes each. This paper also relies on a second mode of data, namely secondary analysis from peer-reviewed journals, popular press, and similarly vetted resources.
In doing this research, we found consistent themes arise, encapsulating ways in which deepfakes could be mitigated, both in a technical and non-technical capacity. Many of these common themes are reflected in the game, The Deepfake Files. However, the game can only provide so much depth and detail on the issues surrounding deepfakes. We also decided that the game was not the appropriate format to delve into more sensitive topics around deepfakes like AIG-CSAM. As such, this brief serves as supplemental material to the game.
A Key Misconception
Throughout our interviews, experts noted a dangerous misconception about deepfake CSAM–that AIG-CSAM is less harmful than non-synthetic CSAM (CSAM that was not created with AI). Our experts unequivocally rejected this claim for a variety of reasons.
First, the child safety ecosystem is already overtaxed. In 2023, the National Center for Missing and Exploited Children (NCMEC) received more than 100 million pieces of suspected CSAM. Trying to sift through the millions of pieces of content and identify children in active abuse scenarios is even more difficult when law enforcement also needs to determine if 1) the content depicts a real child, 2) the identity of a child is concealed with AI, and 3) the depiction of sexual violence is real. AIG-CSAM further strains law enforcement’s limited resources, making it more difficult to save victims of child sexual abuse.
Additionally, malicious actors are fine-tuning models to generate new CSAM of past child sexual abuse targets. New AIG-CSAM of survivors only further victimizes those already dealing with the continuous circulation of original images and videos of their abuse.
Separately, sexual extortion, or sextortion, in the child safety space has grown alarmingly over the past decade. Bad actors will target children, typically young boys, and blackmail them with explicit photos. Now bad actors also blackmail children with AIG-CSAM, using benign photos found on social media. Whether or not these images or videos are “real” is irrelevant to the victims that feel just as violated, isolated, and worried that people won’t believe the content is fake.
The harms of AIG-CSAM are very real.
Closed-Source Models vs Open-Source Models
One of the many factors complicating the prevention of the creation of AIG-CSAM is the difference between closed-source and open-source models. Closed-source models are models where none of their components (i.e. source code or model weights) are publicly available or publicly modifiable, whereas open-source models have one or more components that are publicly available and modifiable. Often, AIG-CSAM is produced with open-source models. In a post on the Substack AI Snake Oil, a group of authors note that open-source models can carry higher risk of misuse because “restrictions on what a model can be used for are both challenging to enforce and easy for malicious actors to ignore. In contrast, developers of closed foundation models can, in theory, reduce, restrict, or block access to their models.”
As one may imagine, the differences between closed- and open-source models can impact mitigation strategies. A strategy that could be effective with closed-source models may not be effective, or possible, with open-source models. For instance, while experts recommend that AI developers and providers track and report inputs and outputs containing CSAM or AIG-CSAM, which many closed-source model developers and providers do, this tracking cannot be implemented with open-source models. Open-source models can be hosted on a user’s own infrastructure with no visibility from the developer or provider.
The scholars cited earlier in this section, along with 20 other authors, published a report in 2024 delving into the risks and benefits of open-source models, proposing a risk-assessment framework for these models. Please note that the debate around closed-source and open-source models is complex and far exceeds the scope of this brief.
Mitigation
Various researchers have conducted in-depth analysis on potential mitigation tactics, targeting both closed-source and open-source models.
The Role of AI Developers & Data Hosting Platforms
Across our interviews, training data, or the content on which machine learning algorithms train, was consistently cited as an area in need of great change. Experts across the board agree that ensuring training datasets do not include CSAM is crucial in preventing models’ ability to produce AIG-CSAM. This is particularly important given a 2023 study by the Stanford Internet Observatory that found CSAM in multiple prominent ML training datasets.
Even without the presence of CSAM, datasets that include benign depictions of children and sexual adult content can also result in models with the ability to produce AIG-CSAM. As such, it’s imperative that developers responsibly source, clean, and intentionally separate content depicting children from sexual adult imagery in their datasets. In Thorn and All Tech is Human’s 2024 report proposing Safety by Design principles to combat AIG-CSAM, they also note the value of sharing already cleaned datasets in an open-source database, when there are no proprietary concerns, to increase access to time- and resource-intensive components.
Models can also be trained to forget concepts, whereby one could train a model to be inept at generating certain topics. There are ongoing discussions on how to responsibly train models on CSAM so that they are unable to produce AIG-CSAM. In a “training to forget” scenario, models would be trained on CSAM to indicate to the models that this is something they should NOT create, which as one may assume carries various ethical and legal questions even for this moral purpose. One scholar also notes the potential value in training models so that they are unable to create children in any depiction, nefarious or not.
Regardless of whether AI developers responsibly source and clean their datasets, stress test their models, or include safety filters, malicious actors in many cases can still fine-tune open-source models and bypass different measures to produce AIG-CSAM. For example, open-source model Stable Diffusion was not only found to be trained on a dataset containing CSAM, but its safety filter was also easily bypassed. While its parent company released an updated version in 2022 that trained on a dataset devoid of any NSFW content, users were frustrated with the quality and restrictions of the output, and the model could still be used by malicious actors for malicious purposes.
In terms of deployment, including provenance markers during the creation of content can help speed up identification. This is particularly valuable given, as previously discussed, law enforcement’s limited resources in trying to identify children in active abuse scenarios. However, provenance and watermarking technologies are still limited and require large buy-in from applicable stakeholders. For a greater discussion on provenance and watermarking, please refer to Make Your Mark (on “Deepfakes”).
Additionally, readers are strongly encouraged to read Thorn and All Tech is Human’s full report on their Safety by Design principles for a more fulsome review of different mitigation tactics at all life cycle stages of AI models.
The Role of Government
On the policy side, there is legal uncertainty around AIG-CSAM and deepfakes more generally. When defining CSAM, the US federal law includes “computer generated images indistinguishable from an actual minor, and images created, adapted, or modified, but appear to depict an identifiable, actual minor.” Additionally, the FBI announced that AIG-CSAM is CSAM in 2024. Stanford Institute for Human-Centered AI policy fellow Riana Pfefferkorn asserts that AIG-CSAM may be criminalized under existing federal law “if either it depicts an actual, identifiable child … or its training data set included actual abuse imagery.” However, she notes that AIG-CSAM that does not fall into the previous categories and is not considered obscene may be protected by the First Amendment. For a more fulsome review of First Amendment protection of AIG-CSAM and Supreme Court precedents, readers should review Pfefferkorn’s whitepaper.
Notably, 20 US states have passed laws criminalizing AIG-CSAM. In most cases, the definition of CSAM was expanded to include AIG-CSAM. While federal law can be interpreted to allow for prosecution of AIG-CSAM, there have been no instances in the US of a federal case being brought solely based on AIG-CSAM. In an interview with nonprofit The Bracket Foundation, digital forensic expert David Haddad assents that “at this point, we have not had a single case that has exclusively considered AI-generated CSAM,” leaving some experts to suggest an explicit legal prohibition of AIG-CSAM at the federal level as a potential solution.
The added obstacle is that AIG-CSAM is somewhat borderless, meaning it can be created, viewed, and distributed across countries. Often, victims are even in different countries than the malicious actors. This makes it difficult to prosecute individuals and provide victims with pathways for recourse. This is also where social media platforms and content moderation are crucial to prevent further proliferation.
The Role of Social Media
While these platforms are able to use detection tools for moderation, they are costly and limited in efficacy. Hash-matching allows platforms to detect known AIG-CSAM. However, particularly with AIG-CSAM, there is such an influx of new content every day that hashing-matching is limited in impact. Tools detecting AI-generated content at large can also be inaccurate, further complicating the issue. Nonetheless, platforms have a legal and moral responsibility to combat CSAM on their sites.
Above all, there are still many questions around the extent of stakeholder responsibility regarding AIG-CSAM–from model developers to social media platforms. Noteworthy, many tech leaders have committed to Thorn and All Tech is Human’s Safety by Design principles in an effort to combat CSAM.
Takeaways
Preventing AIG-CSAM is an incredibly complex issue that involves stakeholders in jurisdictions across the globe and often begs the difficult question of liability. However, it is imperative that stakeholders across the board work to mitigate this heinous misuse of technology. AIG-CSAM, whether or not it depicts real children, endangers children. It will take a multipronged approach across the lifecycle of AI models to protect children.
Please note, this paper does not discuss all potential solutions. We encourage readers to continue to learn about this important topic and read the following sources for additional discourse: Safety by Design for Generative AI: Preventing Child Sexual Abuse, What has Changed in the AI CSAM Landscape, and Generative AI: A New Threat for Online Child Sexual Exploitation and Abuse.
To explore mitigation techniques for deepfakes more broadly, play The Deepfake Files, or dive into a greater understanding of watermarking and provenance in our paper: Make Your Mark (on “Deepfakes”).
Author
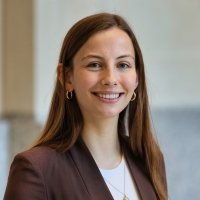
Contributor
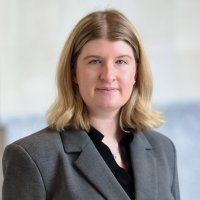
Serious Games Initiative
The Serious Games Initiative communicates science and policy complexities through the world’s most dynamic medium: gaming. Read more
Science and Technology Innovation Program
The Science and Technology Innovation Program (STIP) serves as the bridge between technologists, policymakers, industry, and global stakeholders. Read more
Explore More
Browse Insights & Analysis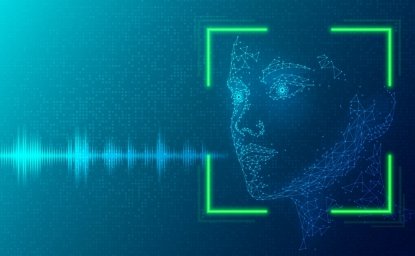
Positive Use Cases of “Deepfakes”
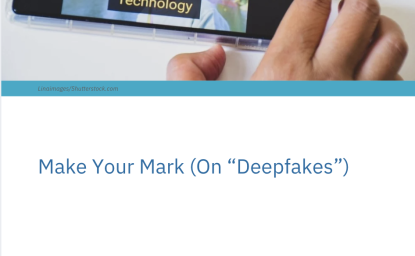